이 포스팅은 인프런 강의 - 선형대수학 개론(조범희)을 듣고 개인 공부를 위해 정리하는 글로, 잘못된 내용이 있을 수 있으니 이 점 참고 부탁 드립니다.
선형대수학개론 - 인프런 | 강의
이 강좌에서는 선형대수학개론을 다루며, 강의를 통해 선형대수학개론을 마스터할 수 있습니다., - 강의 소개 | 인프런
www.inflearn.com
2023.04.16 - [수학/선형대수학] - [선형대수학 개론] 1.3 Vector Equation 에서는 기본적인 벡터 연산과 너무나도 중요한 개념인 Linear Combination 과 Span에 대해 알아봤습니다. 이번 포스팅에서는 행렬방정식(Matrix Equation), $A\mathbf{x}=\mathbf{b}$에 대해 살펴보겠습니다.
[선형대수학 개론] 1.3 Vector Equation
이 포스팅은 인프런 강의 - 선형대수학 개론(조범희)을 듣고 개인 공부를 위해 정리하는 글로, 잘못된 내용이 있을 수 있으니 이 점 참고 부탁 드립니다. 선형대수학개론 - 인프런 | 강의 이 강좌
lotso-land.tistory.com
그럼 포스팅 시작!!!
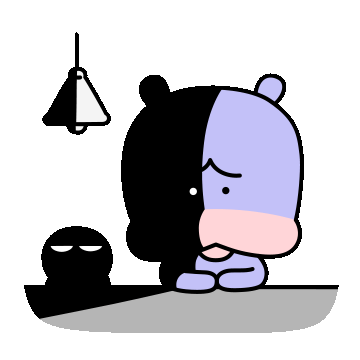
목차
The Matrix Equation
행렬방정식 $A\mathbf{x}=\mathbf{b}$ 에 익숙해지기 위해서 정의와 예제를 살펴보도록 하자.
The Product of Matrix and Vector
matrix $A$와 vector $\mathbf{x}$의 곱 $A\mathbf{x}$ 은 다음과 같이 정의할 수 있다.
For $A_{m\times n}$ with columns $\mathbf{a_1}, \cdots,\mathbf{a_n}$ and $\mathbf{x}\in\mathbb{R}^n$,
$$A\mathbf{x}= \begin{bmatrix} \mathbf{a}_1 \ \mathbf{a}_2 \ \cdots \ \mathbf{a}_n\end{bmatrix}\begin{bmatrix}x_1 \\ \vdots \\ x_n \end{bmatrix} = x_1\mathbf{a_1}+x_2\mathbf{a_2}+\cdots+x_n\mathbf{a_n}$$
$\Rightarrow$$A\mathbf{x}$ is the linear combination of the columns of $A$ using the corresponding entries in $\mathbf{x}$ as weights
Example
- Matrix Equation

- Vector Equation to Matrix Equation

- System of Linear equations to Matrix Equation

More Efficient Way to Compute Matrix Equation

행렬방정식을 풀어쓰지 않고 효율적으로 계산하는 방법은 위와 같다. 즉, $i,1$번째 entry $b_{i1}$는 $A$의 $i$번째 row 와 $\mathbf{x}$ 내적으로 표현된다.

Theorems
[Theorem 3]
For $A_{m\times n}$, with columns $\mathbf{a_1,\cdots,a_n}$ and $\mathbf{b}\in\mathbb{R}^m$,
1. Matrix equation ($A\mathbf{x=b}$)
2. Vector equation ($x_1\mathbf{a_1}+\cdots+x_n\mathbf{a_n}=\mathbf{b}$)
3. Augmented matrix ($\begin{bmatrix}\mathbf{a_1 \ a_2 \ \cdots \ a_n \ b}\end{bmatrix}$)
have the same solution set
- 이는 선형시스템을 각 다른 3개의 관점으로 표현가능함을 의미한다.
[Theorem 4]★★★
For $A_{m\times n}$ (not augmented matrix, i.e., A is a coefficient matrix), with columns $\mathbf{a_1,\cdots,a_n}$ and $\mathbf{b}\in\mathbb{R}^m$,
the followings are all true or all false ;
a. For each $\mathbf{b}\in\mathbb{R}^m$, $A\mathbf{x=b}$ has a solution.
b. Each $\mathbf{b}\in\mathbb{R}^m$ is a linear combination of the colums of $A$.
c. The columns of $A$ span $\mathbb{R}^m$.
d. $A$ has a pivot position in every row.
- 주의해야할 것은 A가 augmented matrix 가 아닌 coefficient matrix 라는 점이다. 만약 augmented matrix $\begin{bmatrix} A \ \mathbf{b} \end{bmatrix}$ 가 pivot position 을 모든 행에서 가진다면, 행렬방정식 $A\mathbf{x=b}$ 는 inconsistent 한 경우가 생긴다. ( $\mathbf{b}$ 가 pivot position 인 경우)
- 주목해야할 것은 a와 d의 동치여부인데, (a)가 참인것의 의미를 이해한다면 쉽게 파악할 수 있다. 이를 이해하기 위해서 2023.02.15 - [수학/선형대수학] - [선형대수학 개론] 1.2 Row Reduction and Echelon Forms에서 공부했던[Theorem 2] Existence and Uniqueness Theorem을 상기시키면 (a)가 참이라는 것은 $\mathbf{b}$가 0이 아닌 $\begin{bmatrix} 0 \ \cdots 0 \ b\end{bmatrix}$ 행이 없는 것을 의미한다. 즉, coefficient matrix $A$가 row reduced 됐을 때, 원소가 모두 0으로 이뤄진 행이 없음을 의미(=최소한 하나의 nonzero 를 원소로 갖는 행들로 이뤄졌음을 의미)한다. 그 이유는 만약 coefficient matrix $A$가 원소가 모두 0으로 이뤄진 행을 가지고 있다면 $b$가 nonzero 인 경우 $A\mathbf{x=b}$는 inconsistent 하고, 이는 $A\mathbf{x=b}$ 가 모든 $\mathbf{b}\in\mathbb{R}^m$ 에 대해 솔루션을 가지는 것은 아니기 때문이다.
[Theorem 5]
If $A_{m\times n}$, $\mathbf{u}$ and $\mathbf{v}\in\mathbb{R}^n$, and $c$ is scalar,then ;
1. $A(\mathbf{u+v})=A\mathbf{u}+A\mathbf{v}$
2. $A(c\mathbf{u})=cA\mathbf{u}$
이번 포스팅은 행렬방정식에 대해 살펴봤다. 다음 포스팅에서는 지금까지 배운 내용들을 바탕으로 선형시스템의 해집합(Solution Sets of Linear Systems)에 대해 살펴본다.
- 오늘보다 나은 블로거가 될 수 있기를 바라며 -
'수학 > 선형대수학' 카테고리의 다른 글
[선형대수학 개론] 1.5 Solution Sets of Linear Systems (0) | 2023.05.11 |
---|---|
[선형대수학 개론] 1.3 Vector Equation (2) | 2023.04.16 |
[선형대수학 개론] 1.2 Row Reduction and Echelon Forms (3) | 2023.02.15 |
[선형대수학 개론] 1.1 Systems of Linear Equations (2) | 2023.02.03 |
댓글